Types of Datasets
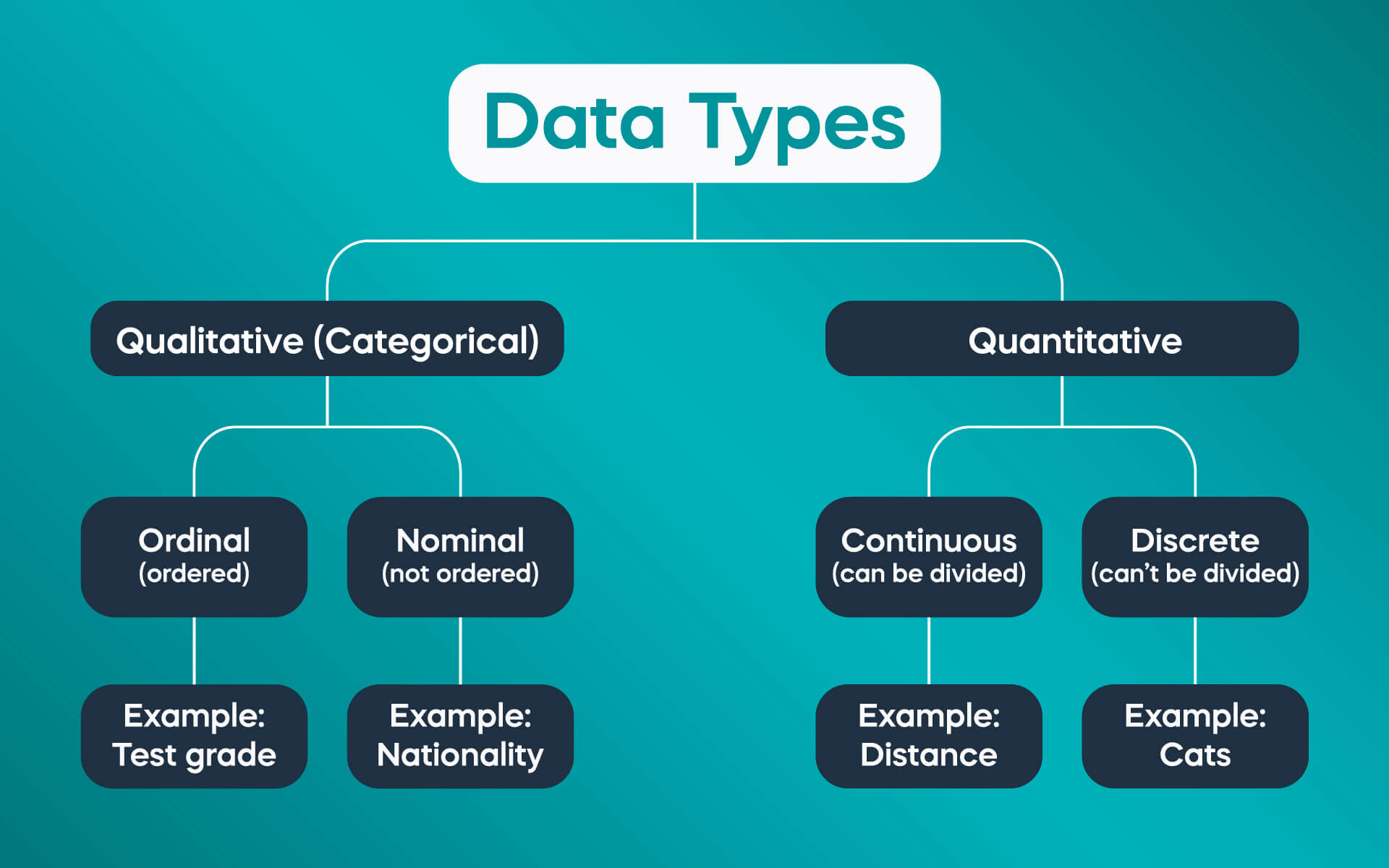
Datasets can vary widely in terms of their characteristics, content, and intended use. Based on these factors, datasets can be categorized into various types. Here are some common types of datasets:
-
Structured Datasets:
- Structured datasets are organized in a tabular format with rows and columns, similar to a spreadsheet or database table. Each row represents a data instance, and each column represents a feature or attribute. Structured datasets are commonly used in machine learning and statistical analysis.
-
Unstructured Datasets:
- Unstructured datasets contain data that does not have a predefined schema or format. Examples include text documents, images, audio recordings, videos, and sensor data. Unstructured datasets often require specialized techniques for analysis and processing, such as natural language processing (NLP) for text data and computer vision for image data.
-
Time-Series Datasets:
- Time-series datasets consist of data points collected at regular time intervals. Each data point is associated with a timestamp, allowing for analysis of temporal patterns and trends. Time-series datasets are commonly used in forecasting, anomaly detection, and trend analysis in fields such as finance, energy, and environmental monitoring.
-
Spatial Datasets:
- Spatial datasets contain geographical or spatial information, such as coordinates, polygons, or geographic features. Examples include maps, satellite images, geographic information system (GIS) data, and location-based data from GPS devices or mobile phones. Spatial datasets are used in urban planning, environmental science, transportation, and disaster management.
-
Text Datasets:
- Text datasets consist of textual data, such as documents, articles, social media posts, emails, and web pages. Text datasets are used in natural language processing (NLP) tasks, including sentiment analysis, text classification, topic modeling, and named entity recognition.
-
Image Datasets:
- Image datasets contain digital images or photographs, often annotated with labels or metadata. Examples include datasets of faces, objects, scenes, medical images, and satellite imagery. Image datasets are used in computer vision tasks, such as object detection, image classification, image segmentation, and image generation.
-
Multimodal Datasets:
- Multimodal datasets contain data from multiple modalities, such as text, images, audio, and sensor data. These datasets integrate information from different sources to provide a more comprehensive understanding of the underlying phenomena. Multimodal datasets are used in areas like multimedia analysis, human-computer interaction, and augmented reality.
-
Relational Datasets:
- Relational datasets consist of multiple tables or data entities linked by common keys or relationships. These datasets are commonly used in relational databases and represent complex relationships between entities in the real world. Relational datasets are prevalent in fields like healthcare, finance, and customer relationship management (CRM).
-
Graph Datasets:
- Graph datasets represent data as a collection of nodes (vertices) and edges (connections) between them. Examples include social networks, citation networks, biological networks, and knowledge graphs. Graph datasets are used in network analysis, social network analysis, recommendation systems, and graph-based algorithms.
-
Transactional Datasets:
- Transactional datasets contain records of transactions or interactions between entities over time. Examples include retail sales transactions, financial transactions, web clickstream data, and social media interactions. Transactional datasets are used in market basket analysis, customer behavior analysis, fraud detection, and personalized recommendation systems.
These are just a few examples of the types of datasets encountered in data science, machine learning, and related fields. Each type of dataset has its unique characteristics, challenges, and analysis techniques, and selecting the appropriate dataset type depends on the specific requirements and objectives of the analysis or application.
Thank you,