Predictive Modeling
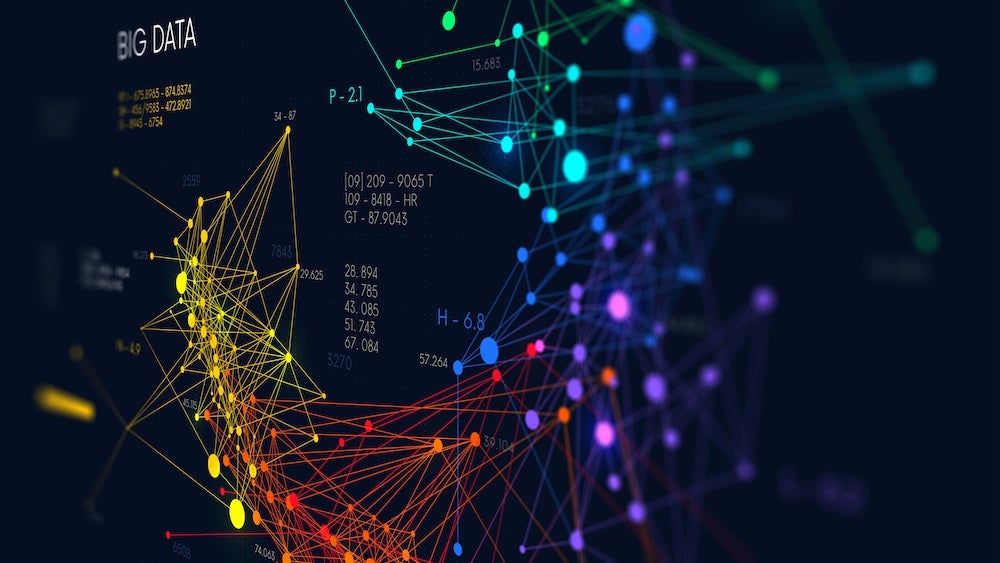
Predictive modeling is a process used in data science and statistics to create a mathematical or computational model that predicts future outcomes based on historical data. The goal of predictive modeling is to identify patterns or relationships within a dataset and use them to make predictions about future events or trends.
Here are the key steps involved in Predictive Modeling:
-
Define the Problem:
- Clearly articulate the problem or question you want to address through predictive modeling. This involves specifying the target variable (the variable you want to predict) and identifying relevant input variables.
-
Data Collection:
- Gather relevant data that can be used to train and validate the predictive model. This data should include historical information about the target variable and associated input variables.
-
Data Preprocessing:
- Clean and prepare the data for analysis. This may involve handling missing values, removing outliers, and transforming variables to ensure they are suitable for modeling.
-
Feature Selection:
- Identify the most important variables (features) that will be used to train the predictive model. Feature selection helps improve the model's accuracy and efficiency.
-
Model Selection:
- Choose an appropriate predictive modeling technique based on the nature of the problem and data. Common techniques include linear regression, decision trees, support vector machines, and neural networks.
-
Model Training:
- Use a subset of the data to train the predictive model. During training, the model learns the relationships between the input variables and the target variable.
-
Model Evaluation:
- Assess the performance of the trained model using a different subset of the data (validation set). Common evaluation metrics include accuracy, precision, recall, and F1 score.
-
Model Tuning:
- Fine-tune the model parameters to improve its performance. This may involve adjusting hyperparameters, choosing different algorithms, or exploring ensemble methods.
-
Deployment:
- Implement the predictive model in a real-world setting to make predictions on new, unseen data. This can involve integrating the model into a software application or business process.
-
Monitoring and Maintenance:
- Continuously monitor the model's performance over time and update it as needed. Data distributions and patterns may change, requiring periodic retraining or adjustments to the model.
Predictive modeling is widely used in various industries, including finance, healthcare, marketing, and manufacturing, to make informed decisions and gain insights into future trends.
Thank you,