Requirement of Data Mining
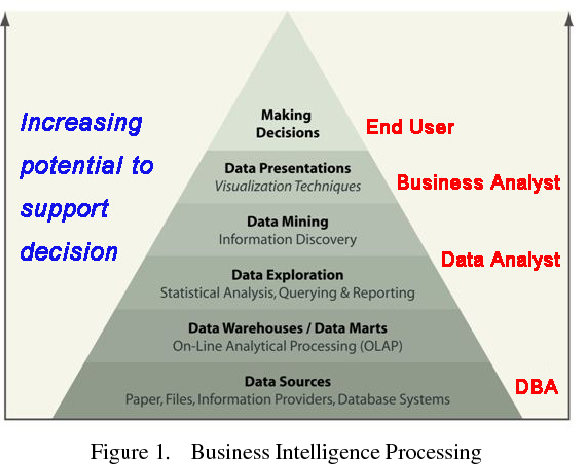
Successful implementation of data mining requires careful consideration of various requirements, including technical, organizational, and domain-specific factors. Here are some key requirements for data mining:
-
Clear Objectives:
- Clearly defined goals and objectives for the data mining project. Understanding what you want to achieve helps guide the selection of appropriate techniques and methods.
-
Quality Data:
- Access to high-quality data is crucial. Ensure that the data is accurate, complete, and representative of the problem you are trying to address. Data quality significantly impacts the results of data mining analyses.
-
Data Integration:
- Integration of data from various sources may be necessary, especially if relevant information is distributed across different databases or systems. Establishing a unified and comprehensive dataset is essential.
-
Data Privacy and Security:
- Compliance with data privacy regulations and the implementation of robust security measures to protect sensitive information. This is particularly important when dealing with personal or confidential data.
-
Domain Knowledge:
- A good understanding of the domain or industry in which the data mining is applied. Domain knowledge helps in selecting relevant variables, interpreting results, and ensuring that the analysis aligns with business goals.
-
Data Preprocessing Tools:
- Tools for cleaning, transforming, and preparing the data for analysis. Effective data preprocessing is essential for handling missing values, outliers, and ensuring the data is in a suitable format for data mining algorithms.
-
Selection of Data Mining Techniques:
- Choosing the right data mining techniques based on the nature of the problem and the goals of the analysis. Different techniques, such as classification, clustering, or regression, may be appropriate for different scenarios.
-
Computational Resources:
- Sufficient computational resources, including processing power and storage, to handle the scale of the data and the complexity of the algorithms. This is especially relevant when dealing with large datasets.
-
Model Evaluation and Validation:
- Techniques and procedures for evaluating and validating the performance of data mining models. It's important to assess how well the models generalize to new, unseen data.
-
Integration with Existing Systems:
- Integration of data mining results with existing business processes and systems. Ensuring that the insights gained are actionable and can be effectively incorporated into decision-making processes.
-
User-Friendly Interfaces:
- User-friendly interfaces for analysts and stakeholders to interact with data mining tools. This facilitates exploration, interpretation, and visualization of results.
-
Scalability:
- Scalable solutions that can handle the growth of data and adapt to changing requirements. This is particularly important in environments where data volumes may increase over time.
-
Training and Skill Development:
- Adequate training and skill development for individuals involved in the data mining project. This includes both technical skills related to algorithms and tools and domain-specific knowledge.
-
Legal and Ethical Considerations:
- Awareness and adherence to legal and ethical considerations, such as consent for data usage, avoiding bias, and compliance with regulations like GDPR.
-
Continuous Monitoring and Maintenance:
- Systems and processes for continuous monitoring of data mining models and regular maintenance to ensure they remain accurate and relevant over time.
Addressing these requirements systematically can contribute to the success of a data mining project and help organizations derive meaningful insights from their data.
Thank you.